Tools for inaccurate human-machine collaborations
Determinist algorithms and systems are based on the expectation of regular and punctual inputs to generate consistent and systematic outputs. Glitchy, uncleaned, misleading or faulty errors in the inputs can lead to suboptimal or erroneous results.
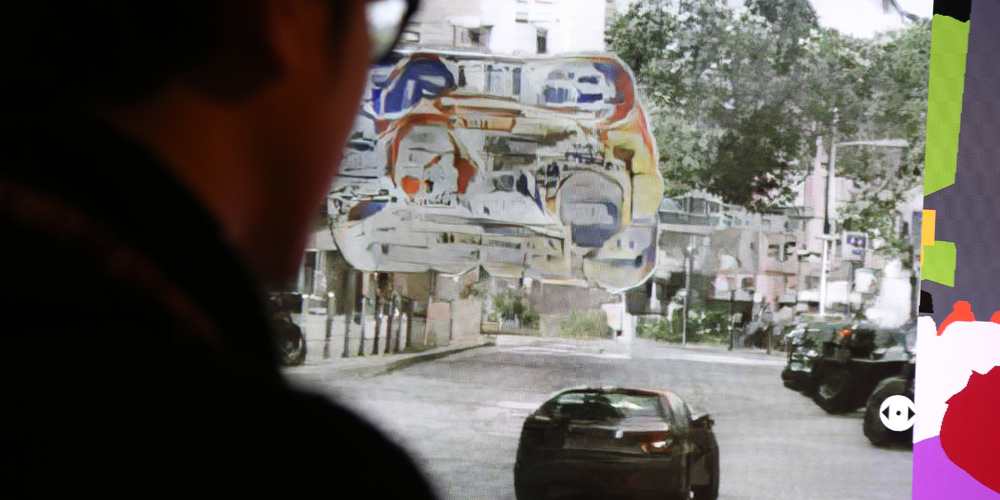
Inaccurate Collaborations is a project that explores how generative and deterministic machine-learning algorithms can be used to create faulty and erroneous results that suggest new explorations for human-machine creative collaborations. Using an interactive digital tool to draw semantic colormap scenes, where each color represents a different label, images are synthesized using Generative Adversial Networks (GAN) that have been trained on a collection of different image datasets. Each generation introduces a new procedural error in the algorithm. The development of this project will make use of RunwayML.
Credits:
RunwayML: https://runwayml.com/
NYU ITP: https://tisch.nyu.edu/itp
Supported by Ministerio de las Culturas, las Artes y el Patrimonio and the Ministerio de Relaciones Exteriores | Gobierno de Chile